現在、yolo v3を使って遊んでいるのですが、そこで自前データを使って学習を行おうとすると学習が始まる直前で
shape_optimizer failed

が出てしまっていたのでその対策を紹介していきます。
エラーの内容
エラーの内容は以下となっていました。
2021-11-21 15:21:51.149144: E tensorflow/core/grappler/optimizers/meta_optimizer.cc:502] shape_optimizer failed: Invalid argument: Subshape must have computed start >= end since stride is negative, but is 0 and 2 (computed from start 0 and end 9223372036854775807 over shape with rank 2 and stride-1)
2021-11-21 15:21:51.192474: E tensorflow/core/grappler/optimizers/meta_optimizer.cc:502] remapper failed: Invalid argument: Subshape must have computed start >= end since stride is negative, but is 0 and 2 (computed from start 0 and end 9223372036854775807 over shape with rank 2 and stride-1)
2021-11-21 15:21:51.353338: E tensorflow/core/grappler/optimizers/meta_optimizer.cc:502] layout failed: Invalid argument: Subshape must have computed start >= end since stride is negative, but is 0 and 2 (computed from start 0 and end 9223372036854775807 over shape with rank 2 and stride-1)
2021-11-21 15:21:51.593370: E tensorflow/core/grappler/optimizers/meta_optimizer.cc:502] shape_optimizer failed: Invalid argument: Subshape must have computed start >= end since stride is negative, but is 0 and 2 (computed from start 0 and end 9223372036854775807 over shape with rank 2 and stride-1)
2021-11-21 15:21:51.627088: E tensorflow/core/grappler/optimizers/meta_optimizer.cc:502] remapper failed: Invalid argument: Subshape must have computed start >= end since stride is negative, but is 0 and 2 (computed from start 0 and end 9223372036854775807 over shape with rank 2 and stride-1)
上記エラーコードの2行目から3行目をグーグル先生に翻訳してもらうと
無効な引数:ストライドが負であるため、サブシェイプは開始> =終了を計算する必要がありますが、0と2です(ランク2とストライド-1のシェイプに対して開始0と終了9223372036854775807から計算されます)
ん~~よくわかりません。
対策
スタックオーバーフローに回答が書いてありました。
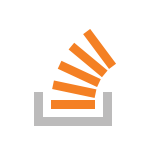
なにやら、yolo3/model.pyに編集を加える必要があるようです。
140~141行目、以下のコードを
box_xy = (K.sigmoid(feats[..., :2]) + grid) / K.cast(grid_shape[::-1], K.dtype(feats))
box_wh = K.exp(feats[..., 2:4]) * anchors_tensor / K.cast(input_shape[::-1], K.dtype(feats))
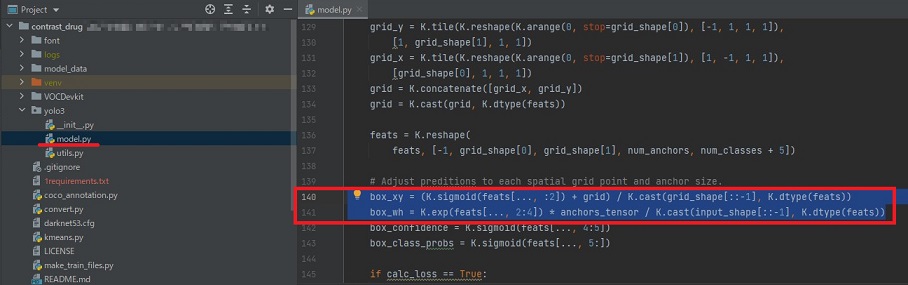
以下のコードに書き換えると解消します。
box_xy = (K.sigmoid(feats[..., :2]) + grid) / K.cast(grid_shape[...,::-1], K.dtype(feats))
box_wh = K.exp(feats[..., 2:4]) * anchors_tensor / K.cast(input_shape[...,::-1], K.dtype(feats))
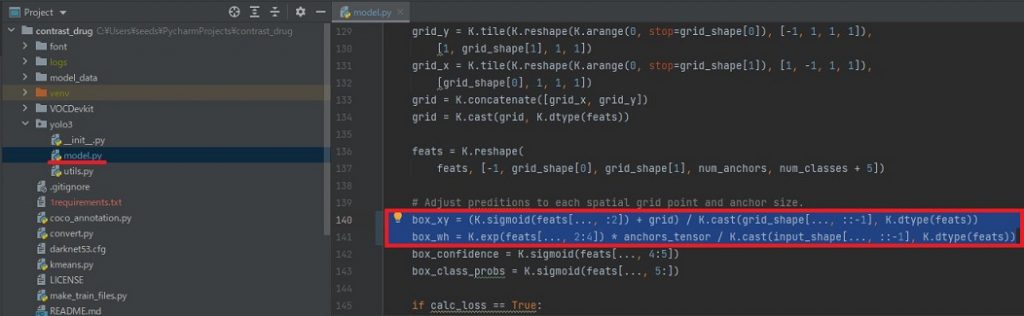
お試しあれ。